Web3: Building a Transactional Chatbot
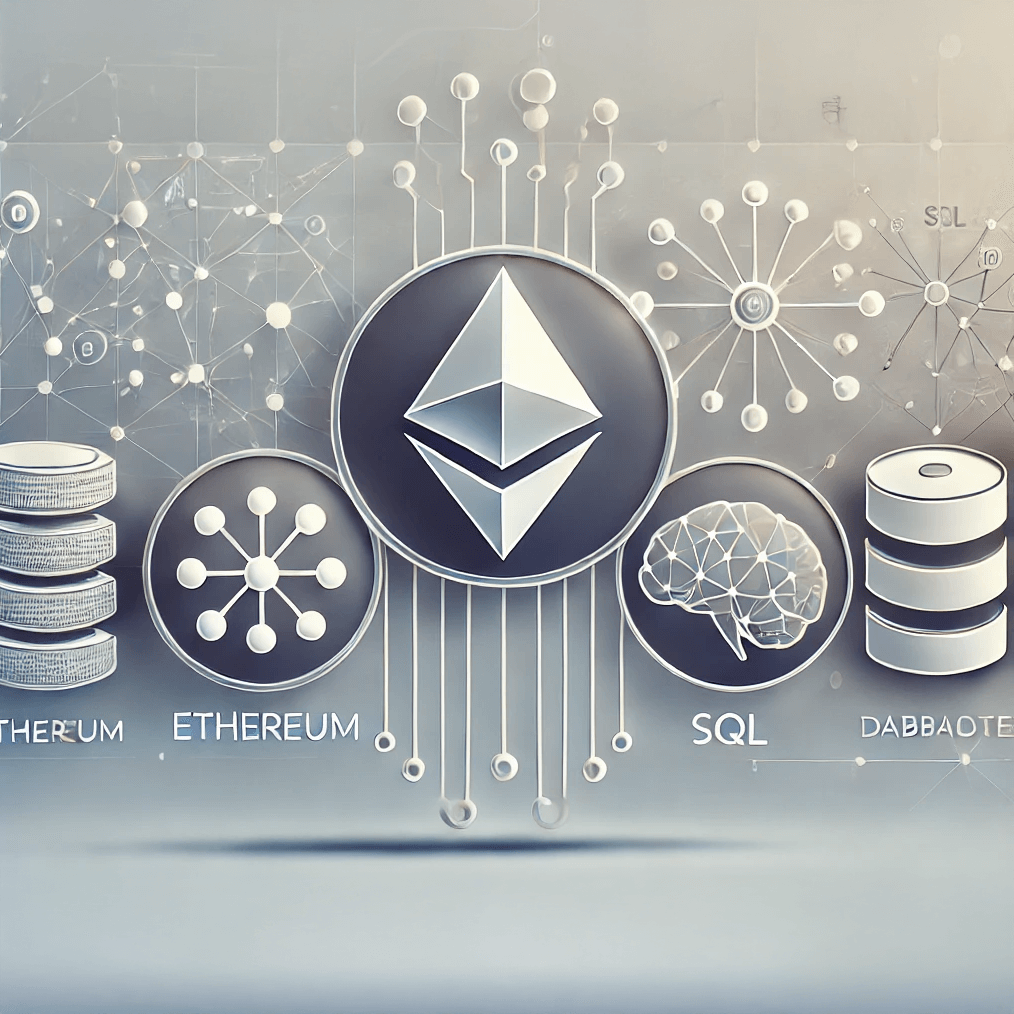
Leveraging Ethereum Data with SQL Agents and AI Introduction In the ever-evolving world of blockchain technology, Ethereum has carved out a unique space not just as a platform for cryptocurrency but as a robust development ecosystem. With its versatile smart contracts and decentralized applications, Ethereum offers a wealth of data that can be harnessed for […]
Empowering Wind Farm Engineers with AI
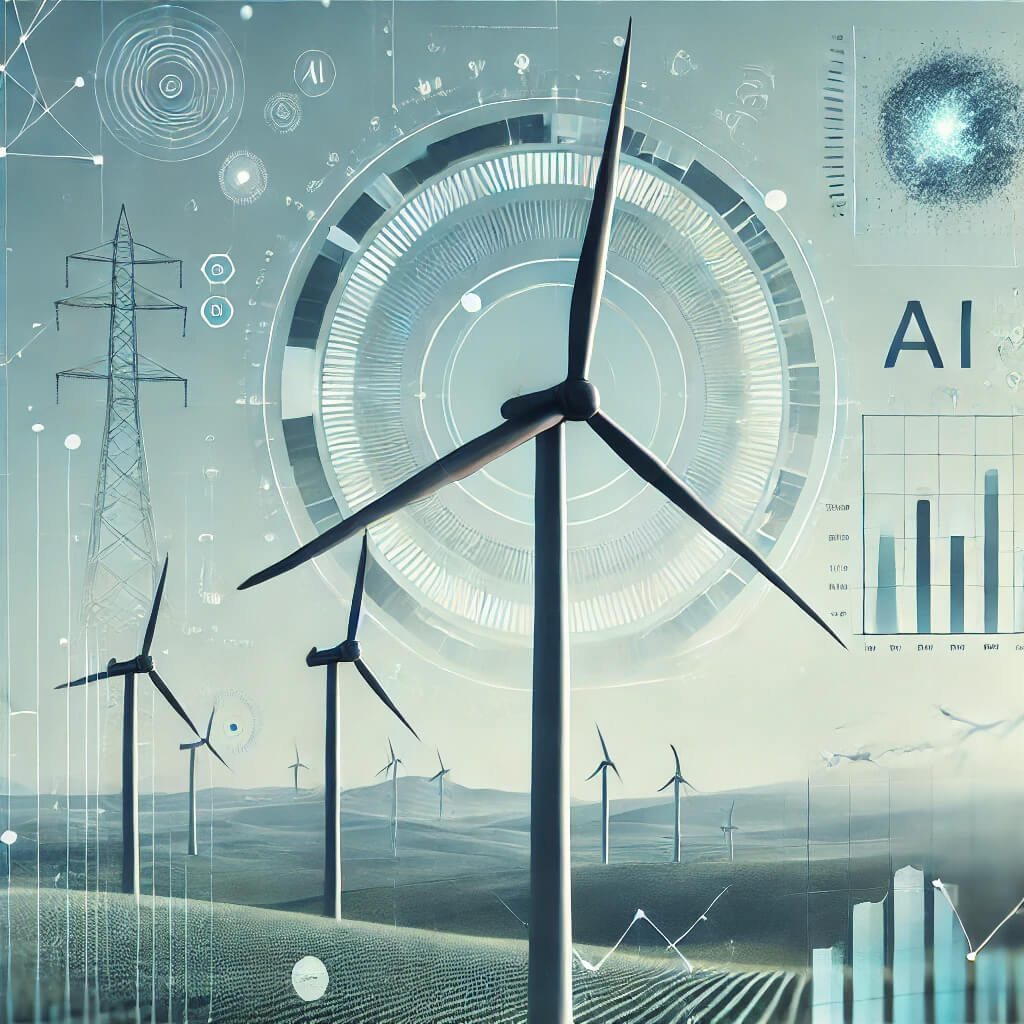
A Hands-On Guide to Choosing the Perfect Turbine Introduction Choosing the right turbine for a wind farm is a high-stakes decision, with the potential to impact everything from energy output to the longevity of the farm itself. Wind farm engineers face a daunting challenge: sifting through countless datasheets, each packed with technical specifications, to find […]
The 10,000-Hour Journey
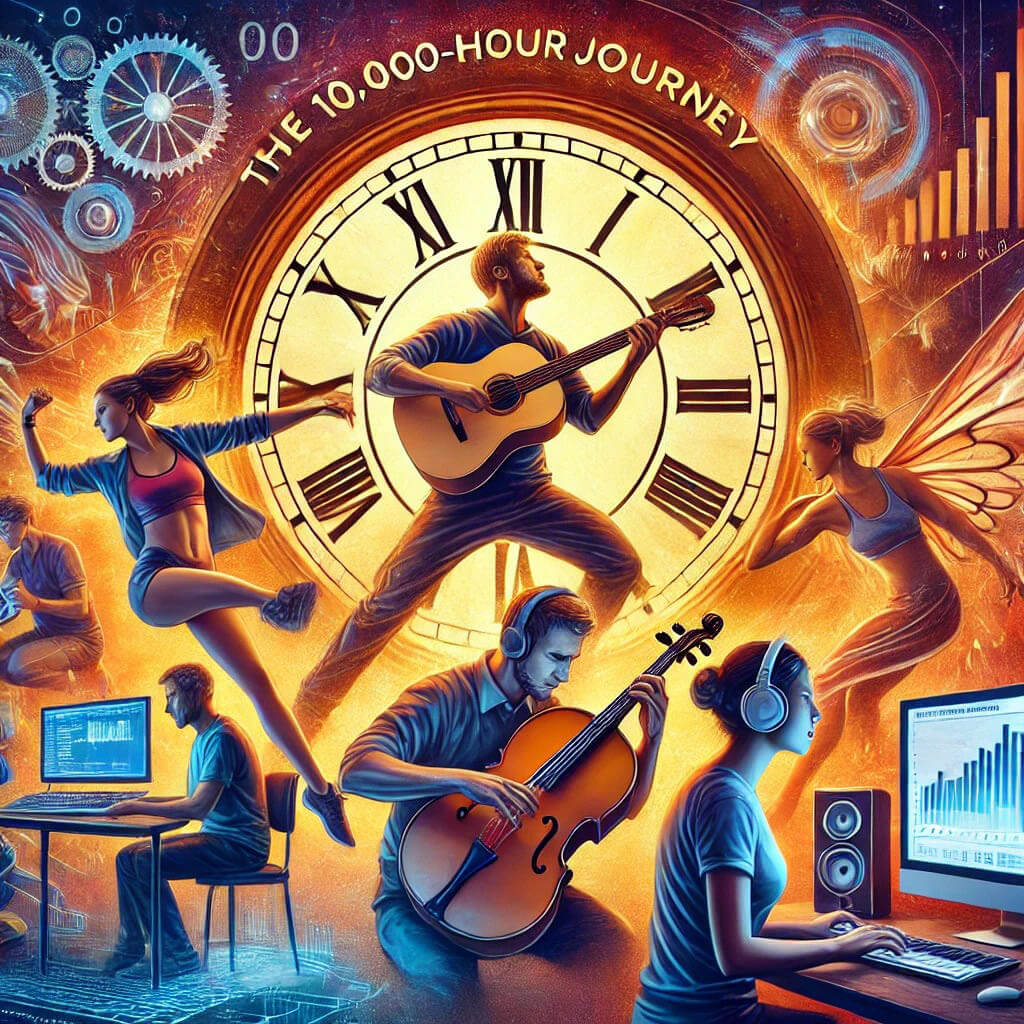
Practice, Perseverance, and the Making of Genius Introduction Imagine a young girl growing up in a poor household, facing numerous challenges and limited resources. She had a passion for music but lacked access to formal training or high-quality instruments. Despite these obstacles, she practiced tirelessly with what she had, often creating makeshift instruments to hone […]
Predicting Telecom Fraud with Linear Regression:
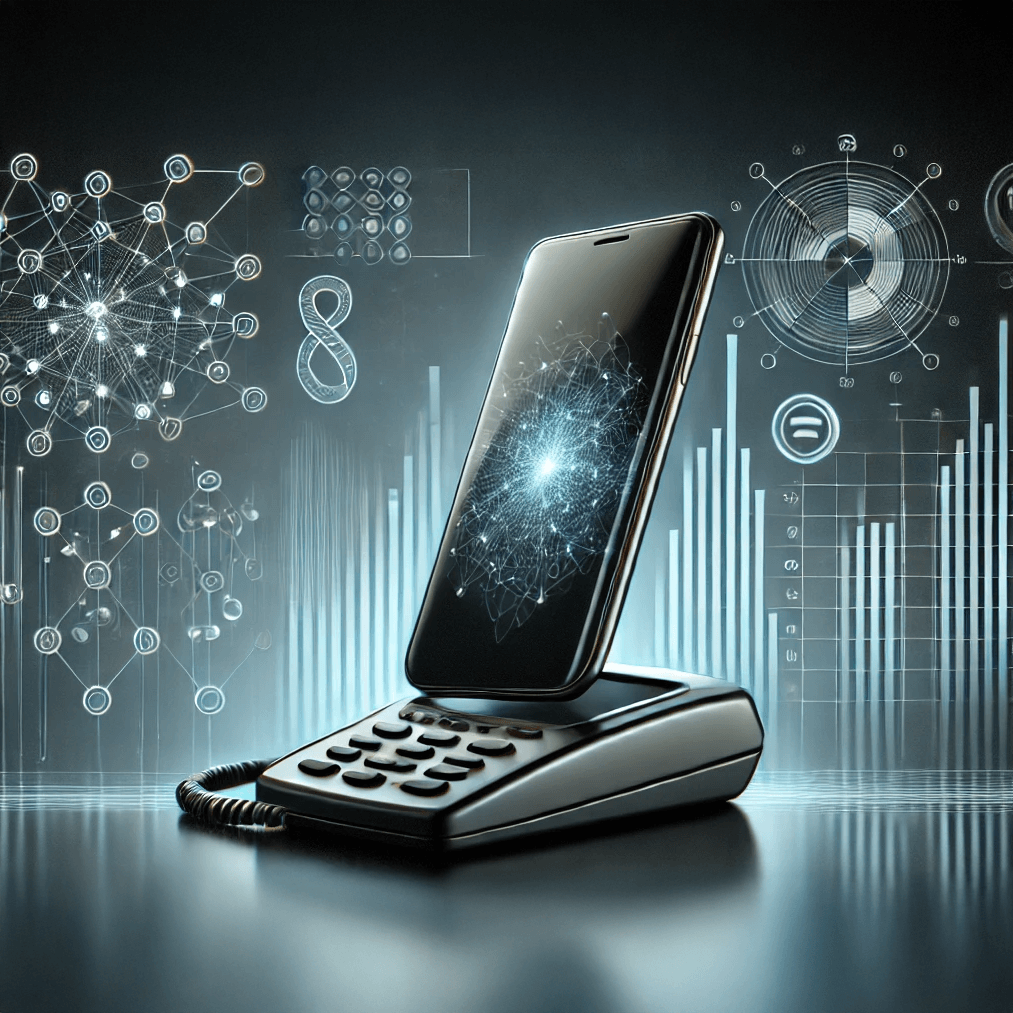
Leveraging Infutor Phone ID and Machine Learning Features Introduction Telecom fraud is a costly and pervasive issue that affects millions of people worldwide. From identity theft to subscription fraud, the tactics employed by fraudsters are continually evolving, making it increasingly challenging for telecom providers to detect and prevent fraudulent activities. In this complex landscape, traditional […]
Python First Look: June 2024 Top Features and Improvements
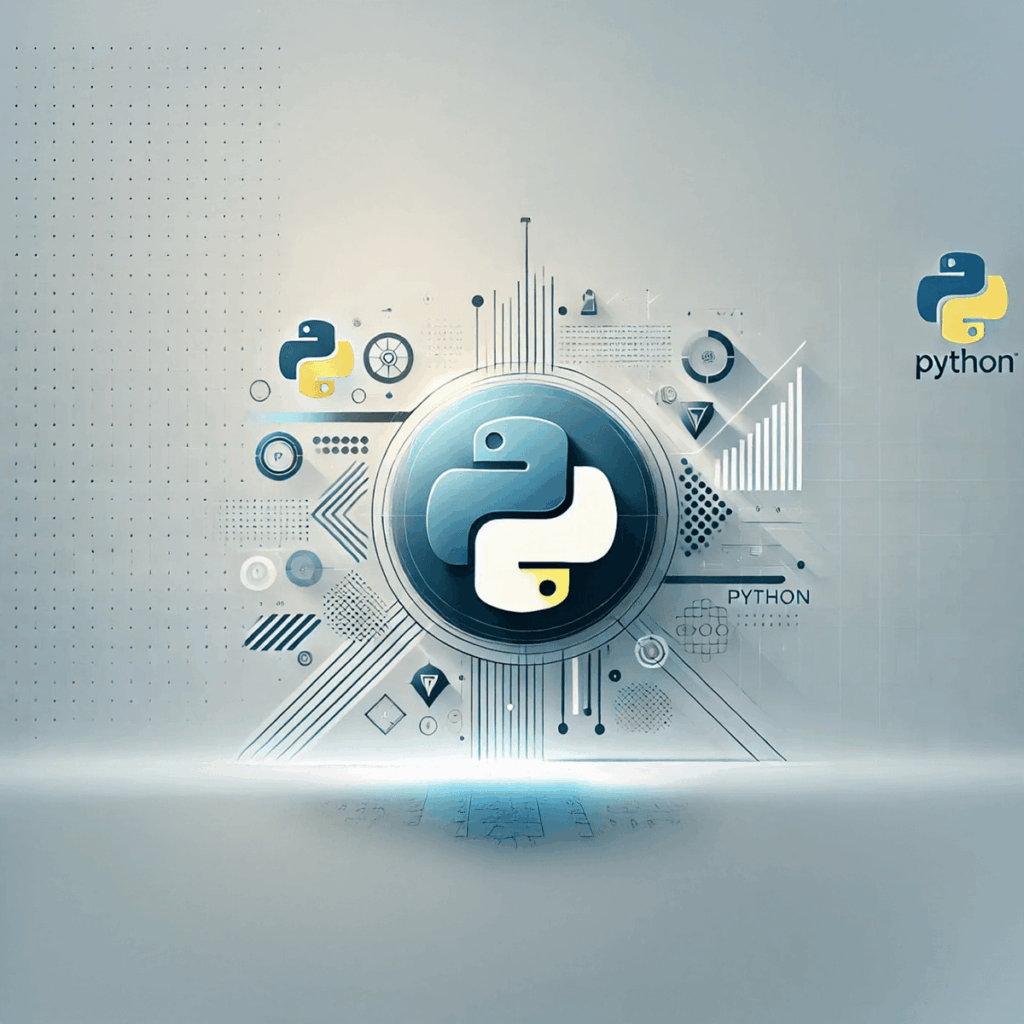
Detailing Python 3.12.4 Release Updates Introduction With the release of Python 3.12.4 in June 2024, the Python community welcomes an array of exciting new features and critical fixes. This version is packed with enhancements that improve security, performance, and usability, solidifying Python’s reputation as a powerful and versatile programming language. Whether you’re a seasoned developer […]
AWS First Look: Key Updates for August 2024
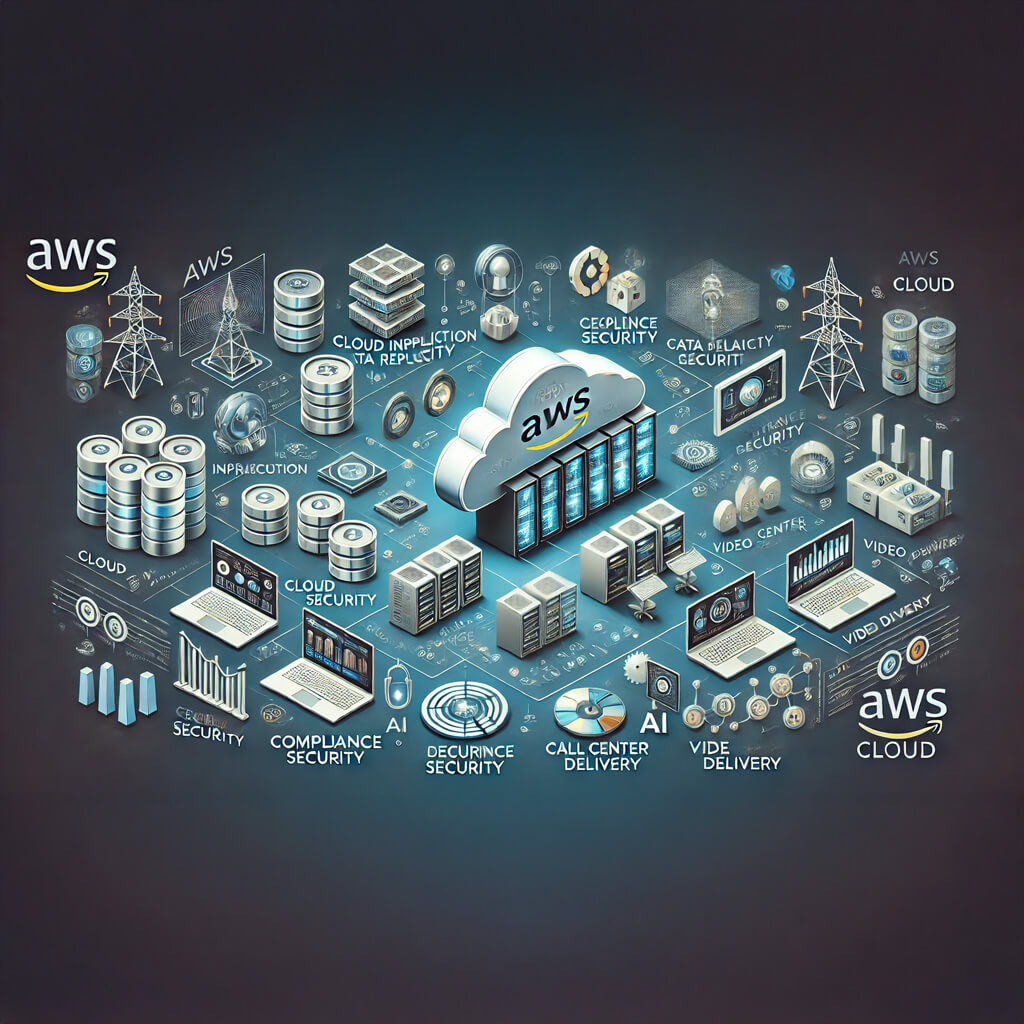
Explore updates to Bedrock FedRAMP, Meta Llama 3.1, SRT Support, and DMS Introduction In the ever-evolving landscape of cloud computing, AWS continues to innovate, delivering new features and updates that enhance the capabilities and reliability of their services. This month brings a host of exciting developments across various AWS offerings. From improved data replication for […]
Balancing ‘Ackchyually’ with Accuracy: Tips for Clear and Kind Communication
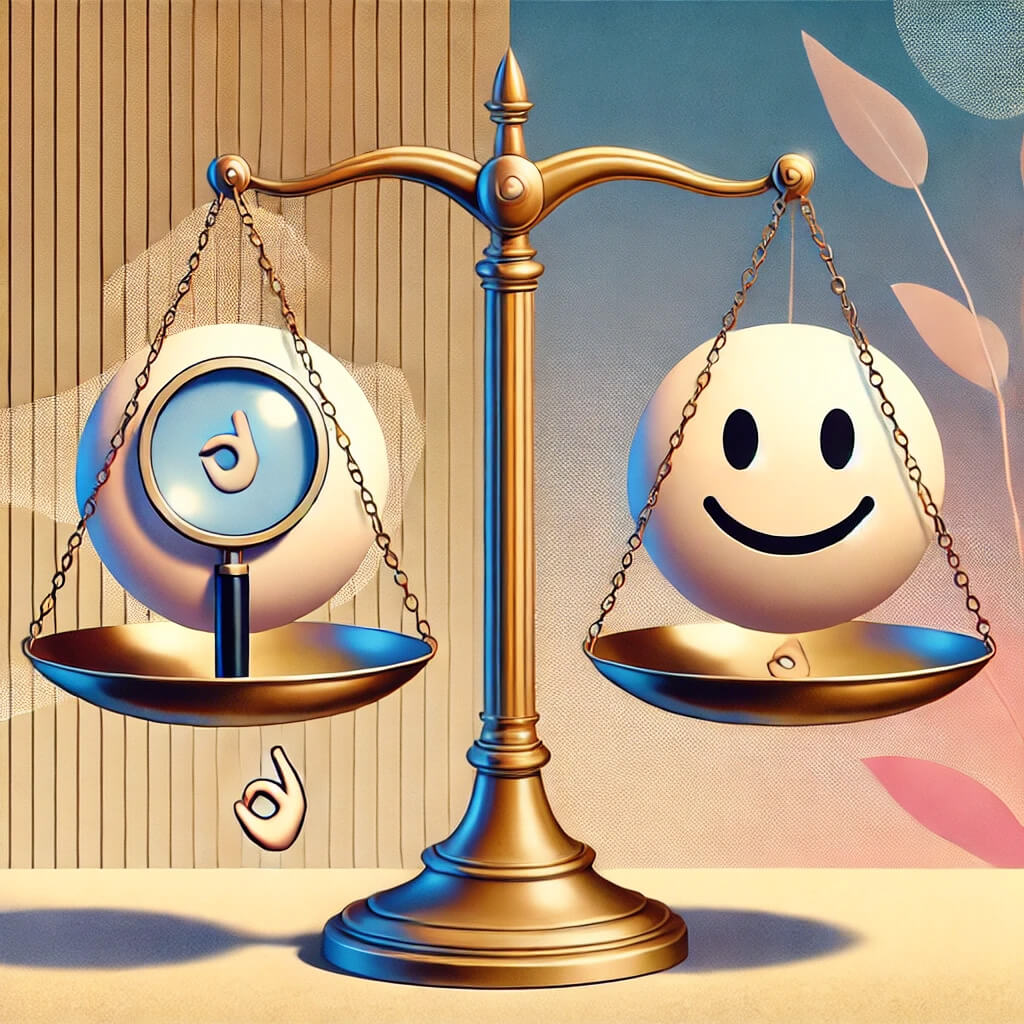
Achieving Precision Without Becoming a Meme Introduction We’ve all encountered the “Ackchyually” guy – that meme-famous character who lives to correct your every word, sometimes missing the forest for the trees. Whether it’s pointing out the difference between “less” and “fewer” or insisting on the exact pronunciation of “GIF,” the “Ackchyually” guy is a reminder […]
Revolutionize Your Development Workflow with VS Code’s June 2024 Features
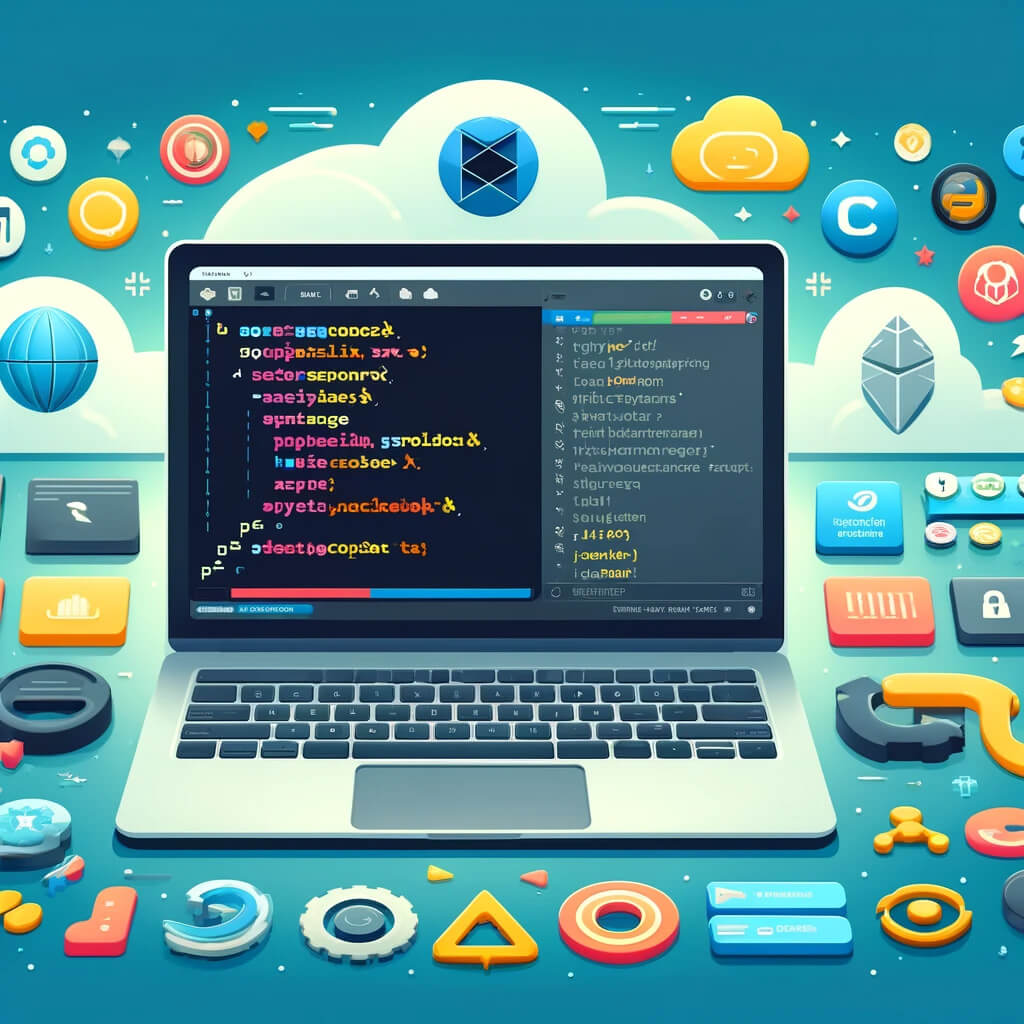
Learn to the exciting new features in the latest VS Code Release Introduction Visual Studio Code (VS Code), known for its versatility and powerful features, continues to evolve, offering developers innovative solutions to enhance their workflows. The June 2024 release of VS Code brings a plethora of updates designed to streamline development processes, boost productivity, […]
Mastering the AWS SA Pro Certification
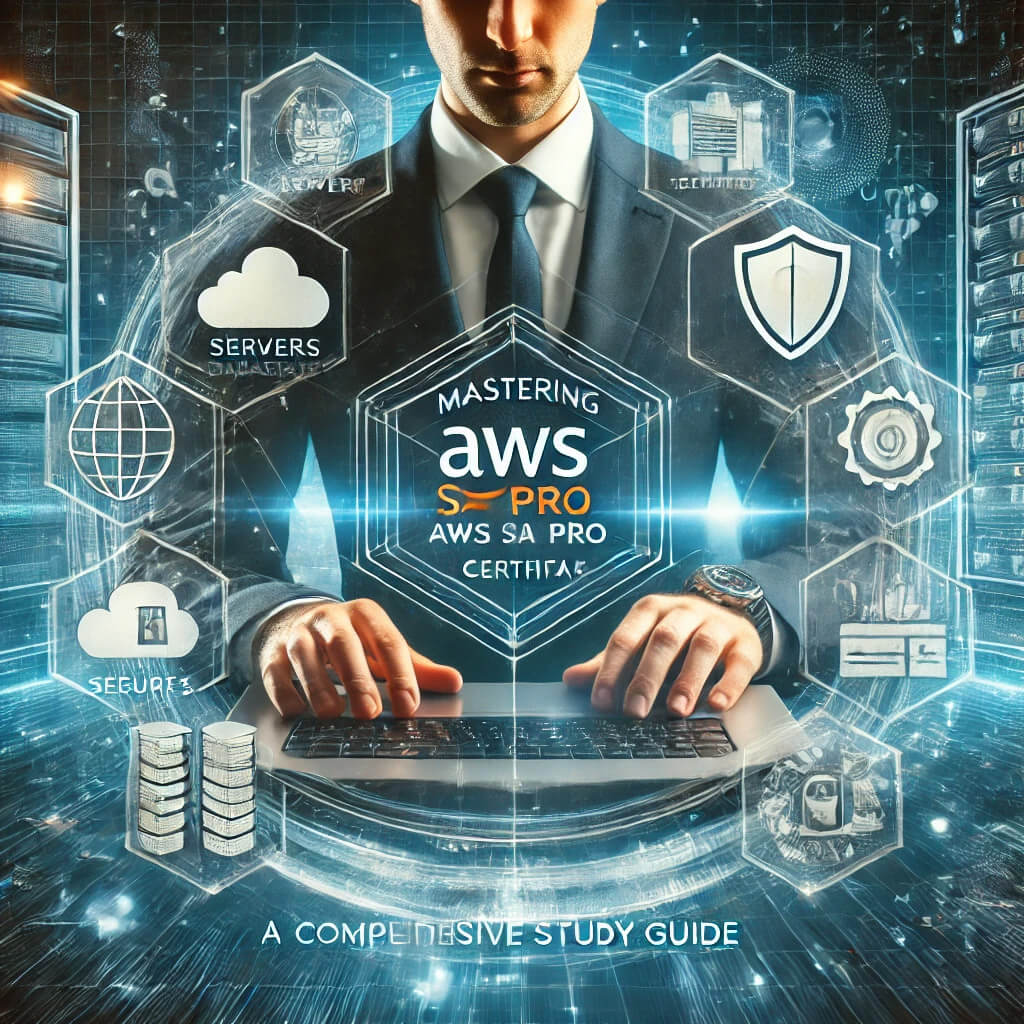
A Comprehensive Study Guide Introduction The AWS Certified Solutions Architect – Professional (AWS SA Pro) certification is one of the most respected and challenging credentials in the cloud computing industry. This certification is designed for individuals with significant experience in designing distributed applications and systems on the AWS platform. Achieving this certification demonstrates a deep […]
Mastering Leadership: Leading by Example, with Compassion, and Through Tough Decisions
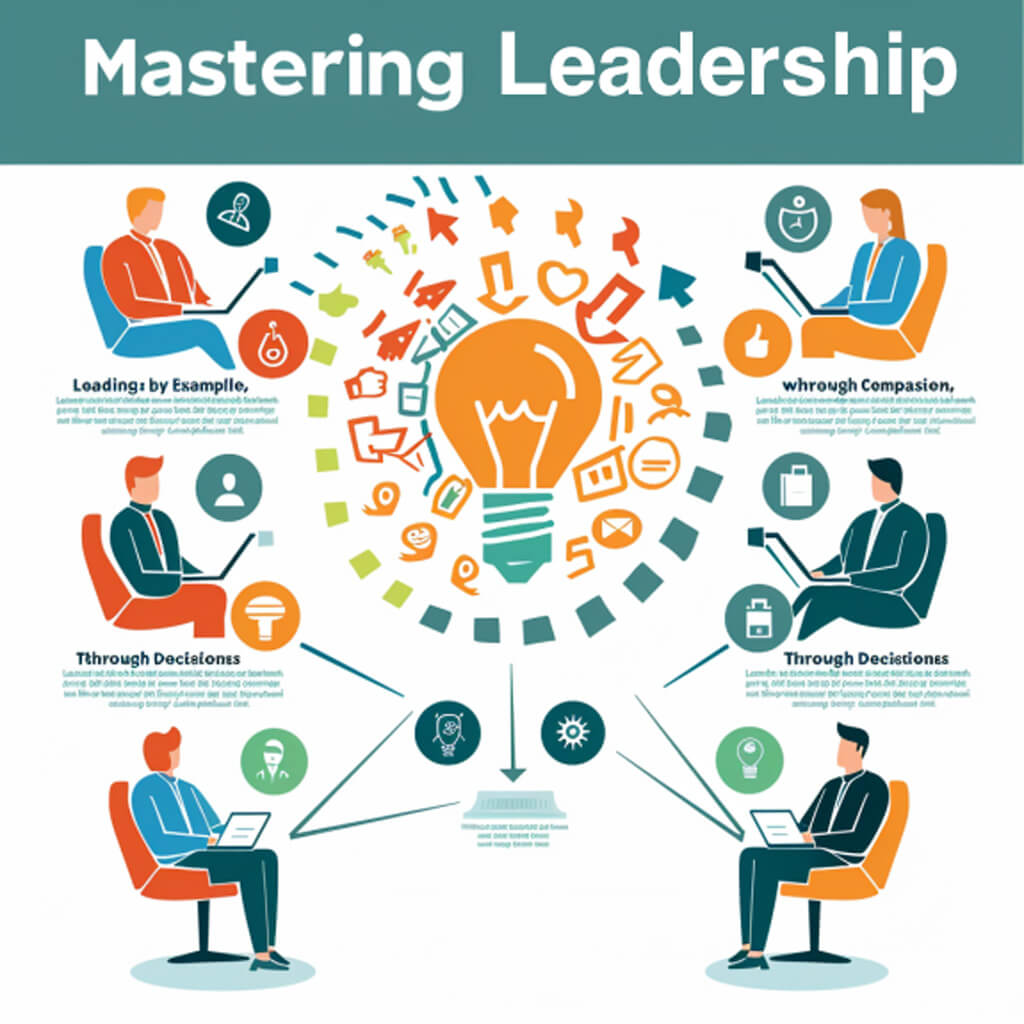
Introduction Effective leadership is a critical component in the success of any organization. The ability to guide and influence a team towards achieving common goals requires a multifaceted approach. This blog post explores three distinct but interconnected leadership styles: leading by example, leading through compassion, kindness, and understanding, and leading when tough choices have to […]