Executive Summary
Customer, leveraging cutting-edge technology in data analytics, partnered with us to overcome challenges in their expansive data collection and processing operations. Tasked with extracting and refining vast datasets from myriad sources, the complexity and maintenance of their system escalated, alongside rising costs associated with data warehousing. Our solution harnessed AI and Language Models to develop a universal extractor, significantly reducing the need for custom coding and streamlining the integration of new data sources. Furthermore, we facilitated a strategic migration from a costly data warehouse system to a cost-effective Data Lakehouse system. This transformation not only optimized operational efficiency and cost but also enhanced agility in onboarding new data points, demonstrating a marked decrease in development and maintenance expenses while accelerating data sharing capabilities.
Customer, leveraging cutting-edge technology in data analytics, partnered with us to overcome challenges in their expansive data collection and processing operations. Tasked with extracting and refining vast datasets from myriad sources, the complexity and maintenance of their system escalated, alongside rising costs associated with data warehousing. Our solution harnessed AI and Language Models to develop a universal extractor, significantly reducing the need for custom coding and streamlining the integration of new data sources. Furthermore, we facilitated a strategic migration from a costly data warehouse system to a cost-effective Data Lakehouse system. This transformation not only optimized operational efficiency and cost but also enhanced agility in onboarding new data points, demonstrating a marked decrease in development and maintenance expenses while accelerating data sharing capabilities.
Customer Description
Founded by experts in the data analytics industry, the customer uses cutting-edge technology and efficient workflow design to collect, curate, and distribute foundational reference datasets.
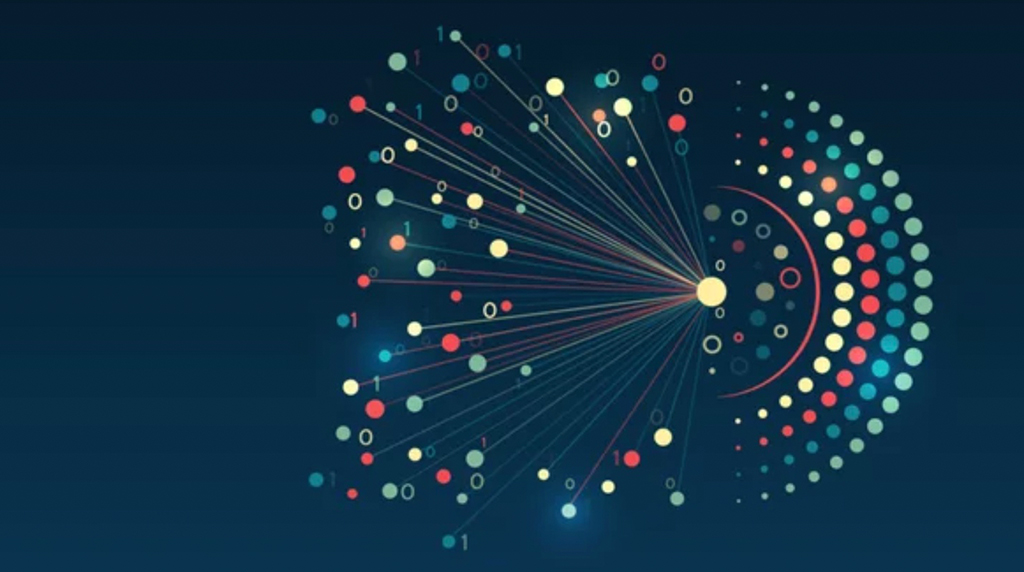