Transforming Financial Services: The Impact of Generative AI
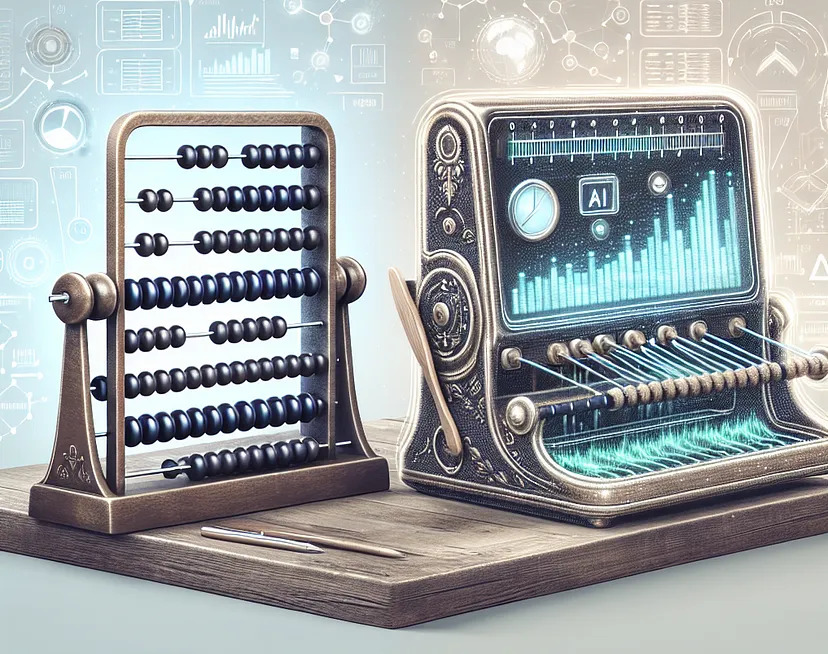
Generative AI is rapidly transforming the FinTech industry, offering groundbreaking solutions that enhance efficiency, bolster security, and elevate customer engagement. This technology, which includes capabilities like natural language processing and machine learning, is being leveraged to automate complex processes, personalize customer interactions, and even combat fraud more effectively. By analyzing vast amounts of data and generating insights at unprecedented speeds, generative AI enables financial institutions to make more informed decisions, streamline operations, and provide tailored services that meet the evolving needs of their customers. As we delve deeper into specific use cases, it becomes clear that generative AI is not just a technological advancement but a pivotal factor in the competitive landscape of financial services.
Anti-Money Laundering (AML) Detection
Generative AI is revolutionizing the way industries tackle complex challenges, including the critical task of detecting and preventing money laundering. In the financial sector, anti-money laundering (AML) efforts are paramount due to both regulatory requirements and the ethical imperative to prevent financial crimes. Generative AI models, particularly those trained on vast datasets of financial transactions, customer behavior, and known fraud patterns, are increasingly being deployed to enhance AML processes.
One of the primary applications of generative AI in AML is the creation of synthetic financial datasets. These datasets help in training machine learning models without compromising sensitive customer information. By using synthetic data that mimics real transaction patterns, financial institutions can improve the accuracy of their AML detection systems without exposing actual customer data.
Furthermore, generative AI can assist in scenario testing and simulation. Financial institutions can use these AI models to generate various potential money laundering scenarios, helping them understand possible vulnerabilities and test the effectiveness of their AML strategies under different conditions. This proactive approach is crucial in adapting to the sophisticated tactics employed by money launderers.
Another significant application is the enhancement of transaction monitoring systems. Generative AI can be used to refine the algorithms that monitor and flag unusual transactions. By continuously learning from new transactions, AI models can become more adept at distinguishing between legitimate activities and potential threats. This reduces false positives, which are a common challenge in traditional AML systems, thereby improving operational efficiency and allowing human analysts to focus on high-risk cases.
Generative AI also contributes to regulatory compliance reporting. By automating the generation of compliance reports with AI, financial institutions can ensure accuracy and timeliness in their filings. This not only helps in adhering to legal standards but also in maintaining trust with regulators and stakeholders.
Overall, the integration of generative AI into AML detection is transforming the landscape of financial security. By leveraging the power of AI to generate data, simulate scenarios, enhance monitoring, and automate reporting, financial institutions are better equipped to combat money laundering effectively and efficiently.
Automated Trading Strategies
Automated trading strategies represent a significant use case of generative AI in the financial sector. By leveraging machine learning algorithms, financial institutions and individual traders can develop models that predict market trends and execute trades at optimal times. These AI-driven systems analyze vast amounts of data, including historical price movements, economic indicators, and market sentiment, to identify profitable trading opportunities.
One of the key advantages of automated trading strategies is their ability to operate continuously and react instantaneously to market changes. This is crucial in a domain where opportunities can emerge and vanish in seconds. Furthermore, these systems minimize human emotional interference, thereby increasing the consistency of trading activities.
Generative AI also facilitates the creation of personalized trading strategies tailored to the specific risk tolerance and investment preferences of users. By processing user performance data and market conditions, AI models can suggest adjustments to trading strategies, optimize portfolio allocation, and propose diversification to mitigate risks.
Moreover, the scalability of AI-driven trading systems allows them to handle an increasing number of assets and data sources without compromising performance. This capability is particularly beneficial for institutions managing multiple assets across different markets.
However, the deployment of automated trading strategies also raises concerns about market stability and the potential for AI-driven market manipulation. Regulatory bodies are thus increasingly focused on developing frameworks to ensure that the use of AI in trading remains transparent, fair, and secure.
Overall, the integration of generative AI into trading strategies is transforming the financial landscape, offering enhanced efficiency and new opportunities for traders and institutions alike. As technology advances, the sophistication and adoption of these systems are expected to grow, further influencing how markets operate.
Fraud Detection and Anomaly Protection
Generative AI is revolutionizing the way industries tackle fraud detection and anomaly protection. By leveraging advanced machine learning models, businesses across various sectors are enhancing their ability to identify and respond to irregular patterns and fraudulent activities more efficiently than ever before.
In the financial services sector, generative AI is used to simulate various fraudulent scenarios, allowing systems to learn and recognize such patterns in transactions. This proactive approach not only helps in catching fraud more quickly but also in reducing false positives, which can be a significant drain on resources. Banks and insurance companies benefit from these AI systems as they can adapt to new fraudulent tactics without requiring explicit reprogramming.
Retail and e-commerce industries also utilize generative AI to safeguard against fraud. AI systems analyze purchasing patterns and customer behavior to flag unusual activities, such as abnormal buying patterns or attempted purchases from previously flagged locations. This capability is crucial during high-volume periods like holidays, where rapid response to potential fraud is necessary to prevent revenue loss and maintain customer trust.
Healthcare is another sector where generative AI plays a critical role in anomaly detection. It helps in identifying unusual patterns in billing, which can indicate fraudulent claims or errors in the billing process. Additionally, AI can monitor patient records to detect outliers in treatment plans or prescriptions, which could signify mistakes or intentional misuse.
The integration of generative AI into security systems extends beyond digital transactions. In physical security, AI algorithms can analyze video surveillance footage to detect anomalous behavior or unauthorized access to restricted areas. This application is particularly useful in critical infrastructure, where security breaches can have severe consequences.
Overall, the application of generative AI in fraud detection and anomaly protection not only enhances the accuracy and efficiency of these systems but also offers a dynamic solution that evolves with emerging threats. This adaptability is key to maintaining resilience in a landscape where fraudulent techniques and anomalies are constantly evolving.
Real-Time Transaction Monitoring
Generative AI has transformative applications in the field of real-time transaction monitoring, particularly within the financial services vertical. By leveraging AI, financial institutions can detect and prevent fraudulent activities as they occur, enhancing security and customer trust.
One of the primary uses of generative AI in this area is the creation of adaptive models that can learn from transaction data in real time. These models are trained on vast datasets of historical transactions, which include both legitimate and fraudulent cases, allowing them to identify patterns and anomalies that may indicate fraud. The AI system continuously updates its understanding as new data comes in, ensuring that it remains effective even as fraud tactics evolve.
Moreover, generative AI can simulate various types of fraudulent behavior to predict how criminals might try to bypass existing security measures. This proactive approach helps banks and other financial entities to strengthen their defenses before new types of fraud are attempted.
Another significant advantage is the reduction in false positives. Traditional fraud detection systems often flag legitimate transactions as suspicious, leading to inconvenience for customers and a higher workload for staff who must verify these transactions. Generative AI improves the accuracy of fraud detection algorithms, reducing the number of false positives and allowing human analysts to focus on more complex verification tasks.
Overall, the use of generative AI in real-time transaction monitoring not only boosts the efficiency and effectiveness of fraud detection processes but also enhances the overall customer experience by minimizing disruptions caused by security checks. As this technology continues to evolve, its integration into daily operations becomes increasingly vital for financial institutions aiming to stay ahead in a digitally driven market.
Credit Scoring and Lending Decision Support
Generative AI is revolutionizing the financial sector, particularly in the areas of credit scoring and lending decision support. Traditionally, credit scoring relied heavily on historical data and linear models, which often resulted in biased or oversimplified decisions. Generative AI, however, introduces a more nuanced approach by leveraging complex algorithms to simulate various borrower scenarios and predict outcomes more accurately.
In credit scoring, generative AI models can synthesize realistic financial profiles based on a wide range of demographic and financial data points. This allows financial institutions to assess risk more dynamically and tailor credit products to individual needs. For instance, by generating synthetic data sets, banks can train their models on diverse scenarios, including rare but potentially significant risk factors that might not be well-represented in real-world data sets.
For lending decision support, generative AI enhances the decision-making process by providing simulations of potential future economic conditions and their impact on borrowers’ ability to repay loans. This predictive capability enables lenders to make more informed decisions, reducing the risk of defaults while also offering fairer terms to borrowers. Moreover, AI-driven tools can automate much of the routine decision-making process, allowing human analysts to focus on more complex cases or strategic tasks.
The integration of generative AI into credit scoring and lending processes not only improves the accuracy and efficiency of financial assessments but also promotes financial inclusion. By better understanding and predicting the financial behavior of underserved groups, institutions can offer more appropriate products and improve access to credit for these populations.
Overall, the use of generative AI in credit scoring and lending decision support is setting new standards for how financial institutions interact with and serve their customers, making the lending landscape more inclusive, predictive, and responsive to individual financial realities.
Customer Segmentation for Targeted Marketing
Generative AI is revolutionizing the way businesses approach marketing by enabling more precise customer segmentation. In the realm of targeted marketing, understanding and categorizing customers based on specific characteristics such as demographics, purchasing behaviors, and preferences is crucial. Generative AI leverages vast amounts of data to identify patterns and clusters within customer bases, which might not be apparent through traditional analysis methods.
For instance, a retail company can use generative AI to analyze transaction data and social media activity to create detailed profiles of potential customer groups. These profiles help in crafting personalized marketing messages that resonate with each segment, thereby increasing the effectiveness of marketing campaigns. The AI can generate simulated customer responses to different messages, allowing marketers to refine their strategies in real-time.
Moreover, generative AI can predict future buying behaviors by simulating how customers might react to changes in products or market conditions. This capability enables companies to proactively adjust their marketing efforts, optimize product offerings, and ultimately achieve higher customer satisfaction and loyalty.
By integrating generative AI into their marketing strategies, businesses are not only able to serve their customers more effectively but also gain a competitive edge by being more agile and responsive to market dynamics. This targeted approach not only enhances customer engagement but also drives better business outcomes through increased conversion rates and ROI on marketing spend.
Personalized Financial Advice
Generative AI is revolutionizing the financial sector by offering personalized financial advice tailored to individual needs and preferences. This technology leverages vast amounts of data, including historical financial transactions, market trends, and personal spending habits, to provide customized recommendations for each user.
One of the primary applications of generative AI in this vertical is in the development of advanced financial advisory bots. These AI-driven advisors can analyze a user’s financial data in real-time and offer insights and advice on a range of financial decisions, from investment strategies to budgeting and savings. Unlike traditional financial advisors, these AI systems can process enormous datasets quickly and provide updates and recommendations instantaneously.
Moreover, generative AI can simulate various financial scenarios based on predictive modeling. This allows users to see potential outcomes of different financial strategies and make more informed decisions. For instance, AI can forecast how different investment choices might perform under various market conditions, helping users to manage risks more effectively.
Another significant advantage is the democratization of financial advice. Generative AI makes high-quality financial guidance accessible to a broader audience, including those who may not afford a human advisor. This technology can help bridge the gap in financial literacy and empower more people to optimize their financial health.
Financial institutions are increasingly integrating generative AI into their services to enhance customer experience and operational efficiency. By doing so, they not only improve customer satisfaction but also gain a competitive edge in the market by offering innovative, personalized financial solutions that meet the evolving needs of their clients.
Predictive Pricing for Financial Products
Generative AI is revolutionizing the financial sector by enhancing predictive pricing models for various financial products. This technology leverages vast amounts of data and advanced algorithms to forecast future price movements more accurately. Financial institutions are using generative AI to simulate different economic scenarios and predict the impacts on prices of stocks, bonds, commodities, and other financial instruments.
One of the primary advantages of generative AI in this vertical is its ability to analyze and interpret complex patterns from diverse data sources, including market trends, geopolitical events, and economic indicators. This capability allows for the generation of detailed pricing models that can adapt to changing market conditions in real-time. As a result, traders, asset managers, and financial analysts can make more informed decisions, enhancing their ability to maximize returns and minimize risks.
Moreover, generative AI contributes to the democratization of financial analysis by providing smaller players with access to sophisticated tools that were once reserved for larger institutions. This shift not only levels the playing field but also promotes a more competitive and dynamic market.
The implementation of generative AI in predictive pricing also extends to personal financial products such as insurance policies and loans. By accurately assessing risk based on a potential customer’s unique data profile, companies can offer more tailored pricing, improving customer satisfaction and loyalty.
As generative AI continues to evolve, its integration into predictive pricing models is expected to become more refined, leading to even greater accuracy and efficiency in financial markets. This progression will likely spur further innovation and adoption across the financial sector, underscoring the transformative impact of AI technologies in this field.
Sentiment Analysis of Financial News
Generative AI has transformative applications across various industries, with the financial sector standing out significantly. One of the most compelling use cases in this vertical is the sentiment analysis of financial news. Financial markets are notably sensitive to news and public sentiment, where even slight shifts in public opinion can lead to substantial market fluctuations.
Generative AI models are adept at processing vast amounts of unstructured data, such as news articles, expert opinions, and social media feeds, to gauge the sentiment conveyed. These models utilize natural language processing (NLP) techniques to understand context, detect nuances, and interpret the sentiment of texts. By analyzing the sentiment of financial news, AI can provide traders, analysts, and portfolio managers with insights that are critical in making informed investment decisions.
For instance, an AI system can continuously monitor different news sources, evaluate the sentiment of financial reports, and alert decision-makers about potential negative or positive impacts on stock prices or market trends. This capability allows for quicker responses to market-moving news compared to traditional methods, which may involve manual data gathering and analysis.
Moreover, sentiment analysis through generative AI can also help in risk management. By understanding sentiment trends, financial institutions can better assess and mitigate risks associated with market volatility. This proactive approach in monitoring and reacting to market sentiments can enhance performance and protect against significant losses.
Overall, the use of generative AI for sentiment analysis in financial news not only optimizes investment strategies and risk management but also provides a competitive edge in the fast-paced financial sector. As AI technology continues to evolve, its integration into financial analytics promises even greater accuracy and deeper insights, further revolutionizing how financial markets operate.
AI-driven Customer Support
AI-driven customer support is transforming how businesses across various industries manage and enhance their customer interactions. By leveraging generative AI technologies, companies are able to provide personalized, efficient, and scalable customer service solutions. This section explores the impact of AI-driven customer support in different verticals.
In the retail sector, AI-driven chatbots and virtual assistants are being used to handle a wide range of customer inquiries, from product recommendations to transaction support. These AI tools can access vast amounts of data in real time to provide customers with accurate information, significantly improving the shopping experience and reducing the workload on human agents.
The finance industry benefits similarly by implementing AI to manage customer inquiries and complaints. AI-driven systems in banks and financial institutions can analyze customer behavior to offer tailored financial advice, detect fraudulent activities, and automate routine tasks like account management and billing inquiries, which enhances compliance and customer trust.
Healthcare is another area where AI-driven customer support is making significant strides. AI applications in this vertical help manage patient data, schedule appointments, and provide non-critical medical advice. This not only improves the efficiency of healthcare providers but also increases patient satisfaction by reducing wait times and improving access to information.
In the telecommunications sector, AI is used to predict and resolve service issues before they affect customers, and to personalize communication strategies to enhance customer retention. The ability to quickly resolve technical issues and proactively manage customer relationships is crucial in this highly competitive industry.
Lastly, the travel and hospitality industry uses AI to enhance customer service by personalizing travel recommendations, automating booking processes, and providing real-time travel updates. This improves the overall customer experience, making travel planning more enjoyable and less stressful.
Conclusion
Across all these verticals, AI-driven customer support tools are not only improving the efficiency of operations but are also enabling businesses to deliver a higher standard of service, tailored to the specific needs and preferences of their customers. As AI technology continues to evolve, its role in customer support will likely expand, offering even more innovative ways to meet the demands of modern consumers.
Generative AI is reshaping the landscape of financial services, offering unprecedented improvements in operational efficiency, risk management, and customer engagement. Embracing these technologies can propel FinTech companies to new heights of innovation and success.
References
- Anti-Money Laundering (AML) Detection
- Automated Trading Strategies
- Detecting anomalies/fraud protection with generative AI
- Real-Time Transaction Monitoring
- Credit Scoring and Lending Decision Support
- Customer Segmentation for Targeted Marketing
- Personalized financial advice based on AI analysis
- Predictive Pricing for Financial Products
- Sentiment Analysis of Financial News
- AI-driven Customer Support